
Melbet ile canlı duyguların ve anında fırsatların dünyasını keşfedin – burada heyecan rahatlıkla birleşiyor ve her an şans getirebilir! Beklemeyin – hemen kaydolun ve kendi şartlarınıza göre oynamaya başlayın!
Melbet ile Türkiye’nin gözde çevrimiçi casino ve bahis portalına erişim
2019 yılından bu yana casino hizmeti sunmaya devam eden Melbet, 7/24 hizmet veren müşteri hizmetleri, %100 Türkçe dil desteği ve güvenilir lisansı ile online casino operatörleri arasında ön plana çıkmakta.
Mobil uyumlu, göz alıcı arayüzü ile oyuncular, %200’e varan Melbet bonuslarından faydalanarak Türkiye ve dünya liglerine bahis yapabilir. Melbet’te bahis severler casino kategorisinde ise Pragmatic Play ve Ezugi başta olmak üzere 25’den fazla sağlayıcı tarafından sunulan oyun seçenekleri ile canlı poker, bakara ve rulet oynayabilir.
Spor bahisleri ve oranları
Melbet, 55’den fazla spor dalı ve 30’dan fazla canlı bahis seçeneği ile oyunculara rekabetçi bahis oranları sunar. Ana sayfadaki “Bahis” ve “Canlı” kategorileri üzerinden bahis yapılması mümkün tüm karşılaşmalar görüntülenebilir.
Oyuncular futbol, basketbol ve voleybol gibi dünya genelinde popüler spor dallarının yanında hentbol, sörf ve satranç gibi alışılmadık alanlara da bahis yapabilir. Melbet ile bahis severler, spor karşılaşmalarında ortalama 1.400 adet gibi yüksek bahis seçeneği arasından özgürce alt/üst bahisleri yapabilir.
Futbol bahis türleri
Türkiye’de en çok takipçisi bulunan spor dalı futboldur. Melbet’te de benzer bir durum söz konusu olup bahis severlerin en çok bahis yaptığı spor dalı futboldur. Oyuncular, Türkiye başta olmak üzere onlarca ülkedeki liglere bahis yapabilir.
Canlı kategorisi altında ofsayt ve korner sayısı, VAR incelemeleri ve topun üst direkten dönme ihtimali gibi tahmin edilmesi zor ancak yüksek oranlara sahip birçok etkinlikle tekli ya da kombine bahisler yapabilirsiniz.
E-spor bahis çeşitliliği
Gençler arasında yaygın takip edilen e-spor turnuvaları yoğun rekabet içeriyor. Milyon dolarlarca ödül dağıtılan turnuvalardaki heyecana Melbet giriş yapıp bahis yaparak ortak olmak mümkün.
CS2, Dota 2, Valorant ve League of Legends başta olmak üzere 10’dan fazla oyunda düzenlenen turnuvalardaki karşılaşmalara bahis yapılabilir. Melbet’te her gün ortalama 180’den fazla e-spor etkinliğine ileri tarihli olarak, 10’dan fazla karşılaşmaya ise canlı bahis imkânı sunulmakta.
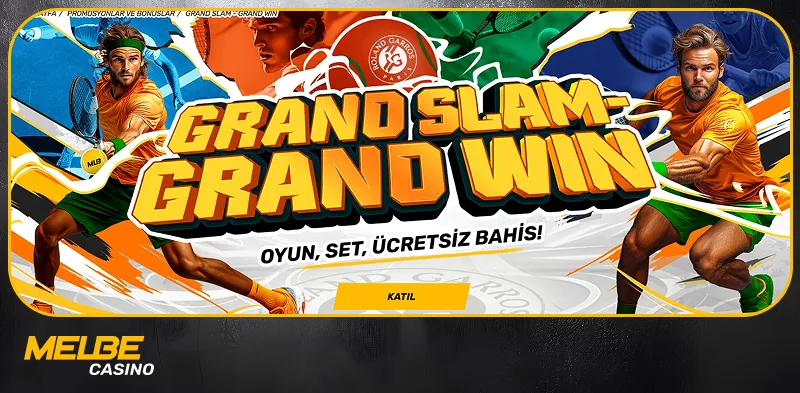
UFC bahisleri
Melbet üzerinden Karma dövüş sanatlarına (UFC) bahis yapabilirsiniz. Oktagon, Glory Kickboxing ve ONE Championship gibi onlarca farklı organizasyondaki sporculara bahis yapabilirsiniz. Karşılaşmaların ortalama 25 dakika sürmesi, pratik bahis severlerin bu spor dalının en sevdiği yönlerden biridir.
Bahis oranları değişken olup dileyen oyuncular canlı, dileyenlerse ileri tarihli bahisler yapabilir. Canlı bahis kategorisi altında oyuncular tahmin etmesi güç ancak bahis oranları yüksek olan, karşılaşmanın hangi raundda bitebileceği ve karşılaşmayı bitirme türüne (KO/TKO) bahis yapabilir.
ŞİMDİ BAHİS YAPMelbet slot oyunları
Riskli ve şansa dayalı olan casino slot oyunları dünya genelinde yaygın bir şekilde oynanıyor. Her oyunun oyuncuya geri dönüş oranı farklıdır. Melbet, bu noktada istatistiksel olarak en kazançlı oyunları ön plana çıkarıyor.
Melbet Casino, 170’den fazla casino oyun sağlayıcıdan binlerce slot oyununun bulunduğu bir kütüphaneye sahip. Türkiye’de en çok oynanan oyun sağlayıcılarından Pragmatic Play, Play’n GO ve NetEnt başta olmak üzere birçok farklı sağlayıcıdan binlerce oyuna buradan ulaşabilirsiniz.
Birçok oyunda var olan demo özelliği ile Melbet oyuncuları gerçek para kullanmadan oyunları test edebilir ve dilerse ardından oyunu masaüstü ya da mobil cihazından oynayabilir.
En tanınan sağlayıcıları ve popüler oyunları şu şekilde sıralayabiliriz:
- NetEnt — Mummy Megaways, Starburst Galaxy ve Piggy Riches
- Pragmatic Play — Gates of Olympus, Big Bass Splash ve Starlight Princess
- Mancala Gaming — Ocean Legacy, Golden Mine ve Coin Craze Jackpot
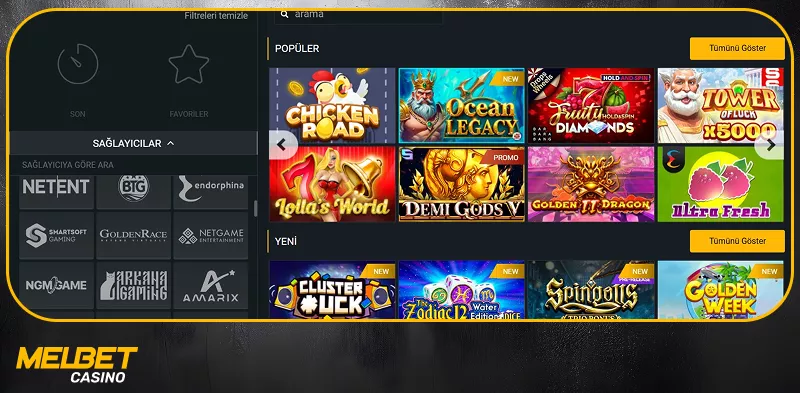
- Barbara Gang — Book of Wealth, Jin Yun Man Man ve Balloon
- Smartsof Gaming — Majestic Wolf, Gold Mine ve Fruity 2 Diamonds
- Manna Play — Neko Cat Fortune, Mariachi Afortunado ve Sweet Dream Bonanza
- MGA Games — Gemblitz Bonanza, Brazilian Carnival ve Hot Fruits on Fire
Melbet bonusları ve promosyonları
Melbet, 25’den fazla bonus ile çevrimiçi casino platformları arasında en yüksek bonus çeşitliliği sunan operatörlerden birisidir. Oyuncular kayıt sırasında spor/casino bahis tercihinde bulunarak ilgi alanına yönelik promosyonlardan faydalanabilir.
Sadakat bonusundan hoş geldin bonusuna, para yatırma bonusundan kayıp bonusuna kadar birçok farklı promosyon türü mevcut. Melbet giriş yapan ve düzenli bahis yapan oyuncular, bonus puanları toplayarak “Promo kod mağazası” içerisinden bonus puanları ile “oranı 1.8 veya daha yüksek olacak olan herhangi bir maça bedava bahis için promosyon kodu” gibi kodları alabilir.
İlk para yatırma bonusları
Bakiyeye ilk kez para yatırıldığında hoş geldin bonusu sunuluyor. Minimum 20₺ maksimum ise 8.000₺’ye kadar yapılan para yatırma işleminde bahis severlerin Melbet hesaplarına %200 bonus tanımlanır.
Melbet’teki bu bonustan faydalanmak isteyenler kayıt olurken spor bahisleri bonus tekliflerine katılmayı seçmeli. Tanımlanan bonus miktarını çekmek için kombine bahislerde toplam 8 kez bahis yapılmalıdır. Her bir kombine minimum 3 veya daha fazla müsabaka içermeli ve bunlardan en az 3 tanesi 2.10 veya daha yüksek oranlarda olmalı.
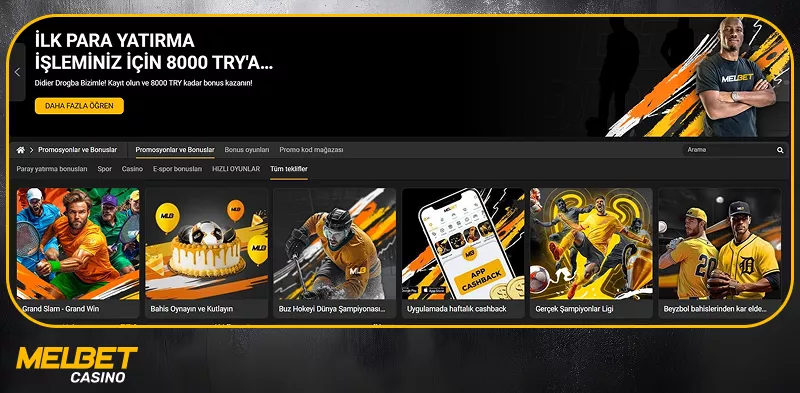
Tekrarlanan para yatırma işlemlerinde sunulan bonuslar
Düzenli para yatırma işlemi yapan Melbet oyuncularına 7’den fazla sadakat bonusu sunuluyor. Türkiye’den Melbet güncel giriş adresi üzerinden giriş yapan tüm oyuncular bu bonuslardan faydalanabilir.
Örneğin ikinci kez para yatıran birisi maksimum 3.400₺’ye kadar yatırdığı tutarının %75’ini bonus olarak kazanır. Üçüncü ve dördüncü para yatırma işlemlerinde bonus %50 ve %25 olarak kademeli düşer. Bonus, 7 gün içerisinde kullanılmalı ve bonus tutarının 5 katı kadar 1,40 veya daha üstü orandan kombine bahis yaparak çevrim şartı yerine getirilmelidir.
Melbet’te büyük talep gören bonuslardan bir diğeri “Kraliyet Pazartesi Bonusu”dur. Oyuncular sadece pazartesi günü yatırdıkları tutar üzerinden 3.914₺’ye kadar %100’ünü bonus kazanır. Bu bonusu almak için gereken minimum para yatırma miktarı 196₺’dir. Bonusu çekebilmek için bonus alındıktan sonraki bir gün içerisinde bonus miktarının 6 katı kadar kombine kuponlara bahis yapma şartı mevcut.
Güncel Melbet casino bonus seçenekleri
Güncel Melbet casino bonus seçenekleri rakip operatörlere nazaran çok daha çeşitli ve kazançlıdır. Casino ve slot oyunları için geçerli bonus kampanyaları özetle aşağıdaki gibidir. Her bonus fırsatındaki şartlar farklı olup detaylı bilgi almak için resmî internet sitesine göz atılması tavsiye edilir.
- Casino hoş geldin kampanyası — Oyuncular, ilk para yatırma işleminde 12.950₺ + 30 FS kazanırken bonus tutarı sonraki yatırma işlemlerinde kademeli olarak artar ve beşinci yatırıma kadar devam eder. Beşinci para yatırımında 12.950₺’ye kadar %200 + 100 FS bonus sunulur. Burada bahsedilen tutarlar maksimum bonus tutarları olup dileyen bahis severler 10€ ile de hoş geldin kampanyasını etkinleştirebilir. Ücretsiz döndürmeler sadece “Juicy Fruits Sunshine Rich” oyunu için geçerli olup çevrim şartı 7 gün içerisinde 40 kattır.
- Sadakat programı — Melbet, 8 farklı aşamadan oluşan bir VIP cashback bonus sistemine sahip. Oyuncular her 1 Euro harcama karşılığında puan toplayarak VIP seviyelerini artırabilir. En alt seviye olan Bakır’da %5 cashback sunulurken Elmas yani son seviyede %15 cashback bonusu sunuluyor.
- Her cuma FS ve bonus ödülleri — Profilindeki kişisel bilgileri dolduran herkes 3.700₺’ye kadar %50 + 100 FS bonustan faydalanabilir. Bonusu almak isteyen oyuncuların her cuma günü hesabına en az 370₺ para yatırması gerekir. Para yatırmadan önce ana hesabınızda bulunan her 185₺ için, Mancala sağlayıcısının “Mariachi Afortunado” oyununda geçerli 1 FS kazanılır. Yapılan para yatırma işlemi üzerinden 3.700₺’ye kadar %50 bonus kazanabilirsiniz.
Ana şart, Melbet’te önceki 6 gün içinde web sitesinde yer alan herhangi bir slot oyununda ve Fast Games kısmında en az 18.500₺ bahis oynamak ve hesabın en az 30 gün önce oluşturulmuş olmasıdır.
Melbet Casino bonuslarına göz attığımıza göre şimdi oyuncuların para yatırma/çekme işlemi için ne gibi seçeneklere sahip olduğunu görelim.
BONUS ALGeçerli para yatırma yöntemleri
Her bahissever kolayca para transfer edeceği yöntemlere sahip olmak ister. Melbet, 62 adet para yatırma yöntemi ile tam olarak bunu sunuyor. Oyuncular dijital banka hesaplarından kripto para birimlerine kadar geniş seçenekler arasından seçim yapabilir.
Tam olarak detaylandırılmış olmasa da Melbet yetkilileri, yüksek tutarlı para yatırma işlemlerinde KYC yani Müşterini Tanı aşamasında oyuncuların kişisel bilgileri doğrulamasını isteyebilir. Melbet giriş yapıp kayıt olarak bu kural kabul edilmiş varsayılır.
Ödeme seçenekleri | Yöntemler | Min. Para Yatırma | Max. Para Yatırma | Süre |
Bankacılık | Cep Bank, Hızlı Havale, Fast, EFT, HayHay Havale, Community Banking, Trink Para Hızlı EFT ve Havale | 50₺ | 100.000₺ | 5-15 dakika |
E-cüzdanlar | PayFix, Payco, Parolapara, PayPay, Jeton Cüzdan, WebMoney, Perfect Money, Sticpay ve AirTM | 11₺ | 250.000₺ | Anında |
Kripto paralar | Binance Pay, Bitcoin (BTC), Litecoin (LTC), Dogecoin (DOGE), Ethereum (ETH), Tether (USDT), Solana (SOL), Dash (DASH) ve Cardano (ADA) | 1 mBTC, 4 mLTC, 2 Doge vb. eşdeğer kripto varlığı | Bilinmiyor | 5-15 dakika |
Kayıt olup Melbet giriş yaptıktan sonra sağ üst köşedeki “Para yatır” düğmesine tıklayarak desteklenen tüm para yatırma yöntemleri görülebilir. Geleneksel yöntemlerden gizliliğin ön planda olduğu kripto varlıklara kadar uzanan para transfer metotlarının belirli aralıkla güncellendiği unutulmamalı.
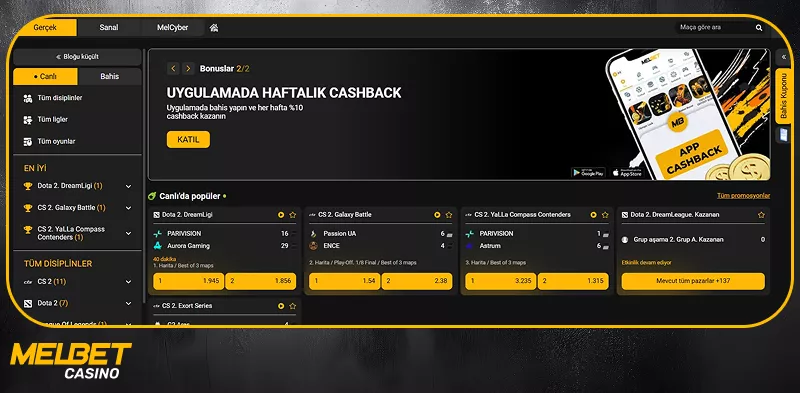
Melbet para çekim süreci
Profiline tıklayıp “Para çek” düğmesine tıklayan Melbet Casino kullanıcıları anlık çekim talebinde bulunabilir. E-cüzdan para çekim talebi saniyeler içerisinde onaylanmakta olup kripto ve bankacılık yöntemlerinde 5-15 dakika arasında sürer.
Küçük tutarlarda yapılacak para çekimlerinde KYC doğrulaması gerekmez ancak yüksek oranlarda para çekmeyi planlayan oyunculardan kimlik doğrulama sürecini başarıyla tamamlamaları istenir. Para çekme işlemlerinin sadece para yatırma işlemlerinde kullanılan ödeme yöntemiyle yapılabileceği de bilinmelidir.
Melbet giriş yapma adımları
Melbet’e ilk kez üye olacak oyuncular, aşağıdaki şu dört basit adımı takip ederek operatöre kolayca kayıt olabilir:
- 1. Adım: Melbet giriş adresini ziyaret et — Resmî Melbet sosyal medya hesaplarından herhangi birisini ziyaret edin. Güncel Melbet giriş adresini öğrenin ve ilgili bağlantıya tıklayın.
- 2. Adım: Kayıt ol — Ana sayfadaki sarı “Kaydol” düğmesine tıklayın. Hoş geldin bonus tercihinizi seçin ve varsa promosyon kodunu girip telefon numarası, e-posta ve şifre gibi kişisel bilgilerinizi doldurun.
- 3. Adım: Doğrulamayı tamamla — Oyuncuların hesaplarını doğrulamak için fatura, kredi kartı ekstresi, kimlik fotoğrafı ve kredi kartı fotoğrafını yüklemesi gerekir. Onay süreci ortalama 1-3 gün sürer.
- 4. Adım: Giriş yap — Ana sayfanın sağ üst köşesinde yer alan “Oturum Aç” düğmesine tıklayıp telefon, e-posta veya SMS bilgisi ile Melbet giriş yapın.
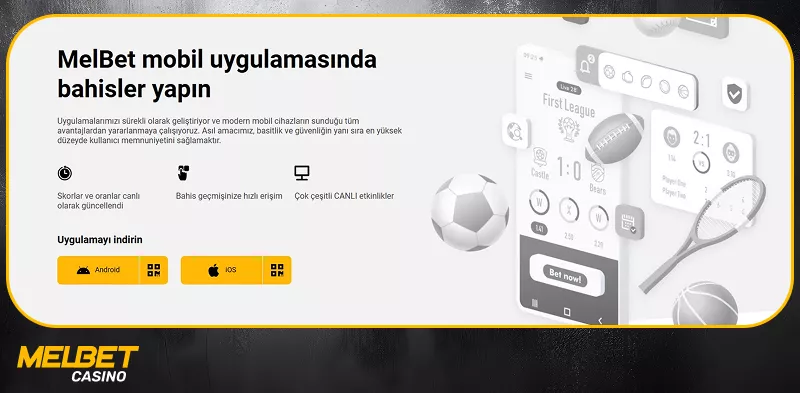
Doğrulama aşamasında beyan edilen bilgilerin doğru olması ve belgelerin içeriğinin net okunması gerekir. Aksi durumda Melbet, oyuncunun hesabını devre dışı bırakma veya silme hakkını saklı tutar.
GİRİŞ MELBETMelbet güncel giriş adresi
Bilgi Teknolojileri ve İletişim Kurumu (BTK), Türkiye’de çevrimiçi casino platformlarına belirli aralıklarla erişim engeli getirmekte. Resmî Melbet sosyal medya hesaplarından oyunculara Bu kısıtlamaların uygulanması halinde resmî Melbet sosyal medya hesaplarından oyunculara anlık bilgilendirme yapılır.
Hemen her gün sahte online casino platformları oluşturulup oyuncular mağdur edildiğinden oyuncular güvenliklerine dikkat etmelidir. Melbet güncel giriş adresi Telegram ve X (eski adıyla Twitter) başta olmak üzere resmî kanallarından takip edilebilir.
Dileyen bahis severler Melbet giriş adresini öğrenmek adına canlı destek ya da destek e-posta üzerinden bağlanarak teyit amaçlı müşteri temsilcilerine danışabilir.
Casino Melbet güvenilirliği
Melbet, Antillephone adlı dünyanın en itibarlı casino lisanslarından birine sahiptir. Sahip olduğu Curaçao lisansı ile oyuncular düzenli denetlenen bir ortamda güvenle bahis yapabilir. OGL/2024/561/0554 lisans numarası sorguya açık olup dileyen bahis severler Melbet giriş yapmadan önce teyit edebilir.
Online casino sektöründe sahte casino operatörler yaygındır. Lisansa sahip olmayan casino sitelerinin AML/KYC kurallarına tabi olmaması nedeniyle oyuncular dolaylı yoldan yasa dışı suçlara ortak olabilir. Araştırmalar, Melbet güvenilir mi sorusuna güçlü lisansı, 500’den fazla aktif çalışana sahip olması, şikâyet oranlarının minimal düzeyde yer alması ve uzun yıllardır bu sektörde olması sebebiyle “güvenli” yanıtı verildiğini göstermekte.
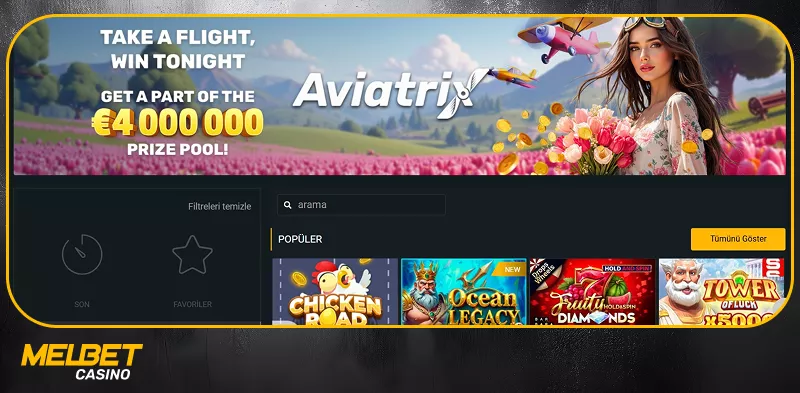
Melbet mobil indirme süreci
Melbet mobil indirme süreci son derece basittir. Melbet APK sürümünü indirmek için resmî Melbet giriş yapmalı ve ana sayfanın sol üst bölümünde yer alan “Application” düğmesine tıklamalısınız.
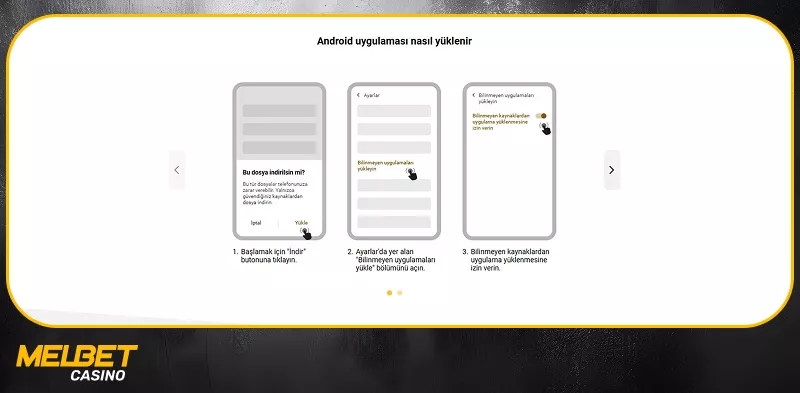
Ardından açılan sayfadan “Android” ya da “iOS” simgesine tıklayıp uygulamayı indirmeye başlayabilirsiniz. Eğer indirme sürecinde hata alırsanız telefon ayarlarından “”Bilinmeyen kaynaklardan uygulama yüklenmesine izin verin” seçeneğini etkinleştirmeyi unutmayın.
İNDİRMEKMelbet APK ve iOS sürümü
Melbet, mobil uyumlu internet sitesine ve harici bir resmî uygulamaya sahiptir. Mobilden Android ve iOS uygulamasını indirmek için oyuncular bazı gereksinimleri karşılaması gerekir. Aşağıdaki bölümde platforma göre Melbet indirme gereksinimleri bulabilirsiniz:
- Android uygulaması — Play Store’daki kısıtlamalar nedeniyle Melbet APK uygulaması yalnızca web sitesinden indirilebilir. İndirilecek olan cihazın minimum Android 4.1 veya daha yüksek bir sürüme, 1 GB RAM ve 100 MB boş alana sahip olması gerekir.
- iOS uygulaması — App Store’dan indirilebilir ve iOS 12 veya daha yüksek sürüme sahip cihazlar için uygundur. 1 GB RAM ve 178 MB depolama alanı gerektirir. Ayrıca, mağazadan erişilebilmesi için Apple ID konumu değiştirilmelidir.
Mobil üzerinden casino oyunları veya spor bahisleri konusunda herhangi bir kısıtlama yoktur. Oyuncular dilerse uygulama üzerinden dilerse de mobil uyumlu Melbet tarayıcısını ziyaret ederek tüm oyun kütüphanesine erişebilir.
Sunulan binlerce casino oyununun yanında spor bahisleri kategorisi altında Türkiye liglerinin de arasında bulunması Melbet’i benzerleri arasında ön plana çıkarıyor. %100 güven mottosuyla hizmet veren Melbet, kullanıcılarının verilerini SSL sertifikası ve 256 bit şifreleme protokolü ile koruyor.
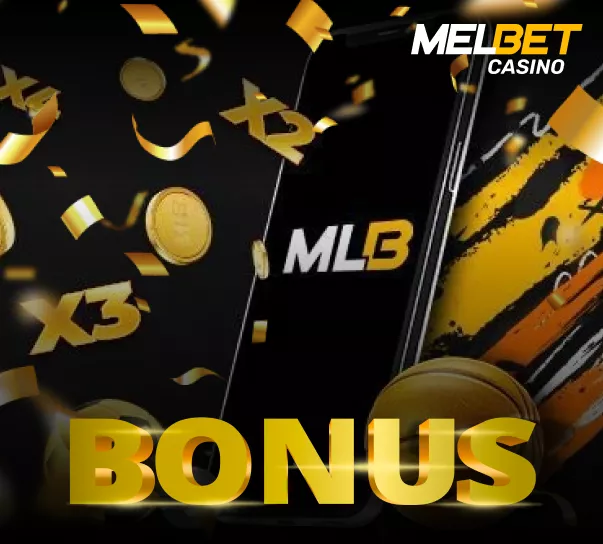
